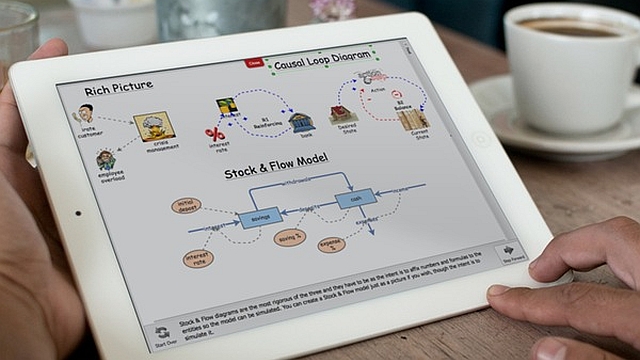
Models and Truth: Summary [Systems thinking & modelling series]
This is part 38 of a series of articles featuring the book Beyond Connecting the Dots, Modeling for Meaningful Results.
Now that we have thoroughly described the concepts of narrative and predictive models we can conclude the “Models and Truth” section by taking a step back and reemphasizing that these two categories do not represent specific modeling techniques. You can build a stock and flow model to tell a story about a system resulting in a narrative model. If your story of the system accurately represents how the system operates in reality, then you will also have a model that generates accurate predictions.
Similarly, you can apply a linear regression to a dataset. If the relationship in the data is truly a completely linear one, then the result of this regression will be the most accurate predictive model you could build. On the other hand, if you do not assess the predictive accuracy of the model and just use a linear regression because it is easy to interpret or because it matches you understanding of reality, then you have a narrative model.
The key criteria to remember when building your own models or assessing other people’s models is that a predictive model is one for which you have an accurate assessment of the errors of the predictions. A good predictive model is one that has low relative errors when compared to other predictive models for the same system. A narrative model is one that tells a story about the system. A good narrative model is one that persuades an audience, and by persuading, the model transforms the mental models of its audience.
Next edition: Building Confidence in Models: Introduction.
Article sources: Beyond Connecting the Dots, Insight Maker. Reproduced by permission.
Header image source: Beyond Connecting the Dots.