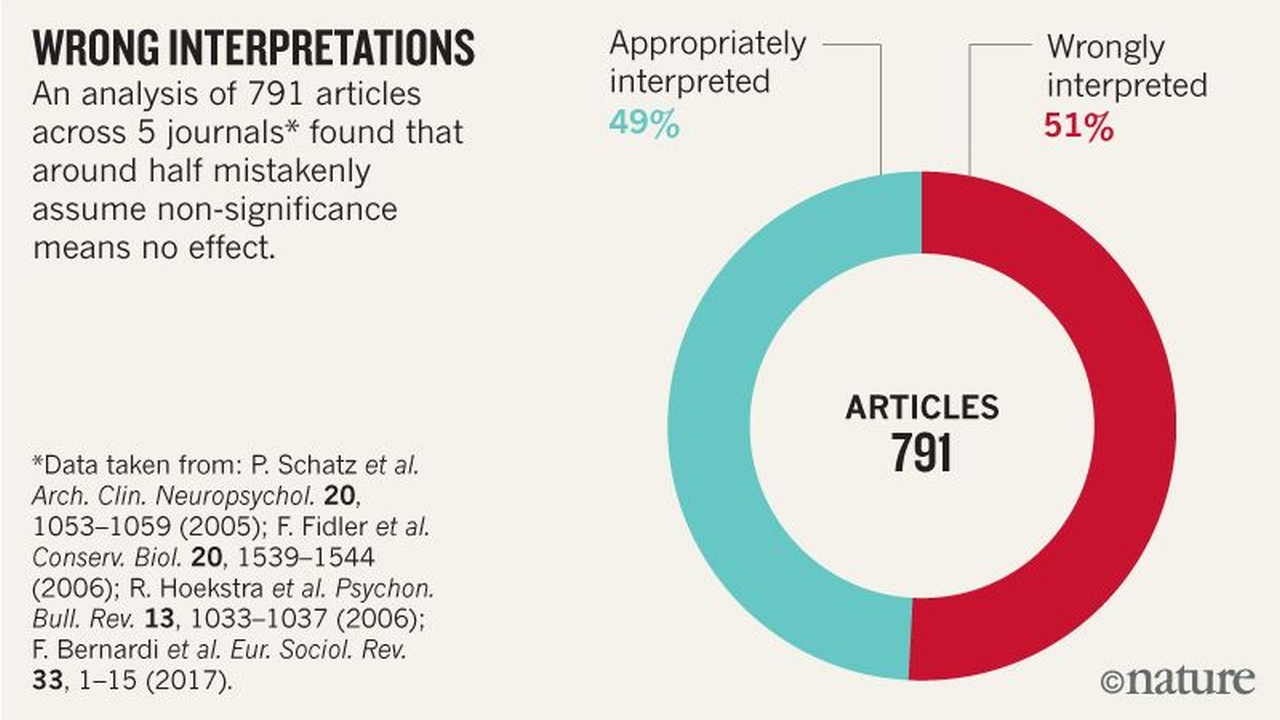
Scientists rise up against statistical significance [Top 100 journal articles of 2019]
This article is part 3 of a series reviewing selected papers from Altmetric’s list of the top 100 most-discussed scholarly works of 2019.
As we’ve previously reported in RealKM Magazine, the American Statistical Association (ASA) released a statement1 in 2016 warning against the misuse of statistical significance and associated calculated probability values (p-values). The statement included six principles underlying the proper use and interpretation of the p-value.
In March this year, the ASA sought to push these reforms further through the publication of a special issue of their journal, The American Statistician. This prompted over 800 scientists in more than 50 countries to sign a comment calling for statistical significance to be retired, bringing an end to hyped claims and the dismissal of possibly crucial effects.
The comment was published in the journal Nature, subsequently becoming the #2 most discussed article2 in Altmetric’s top 100 list for 2019. The authors of the statement advise that:
We are not calling for a ban on P values. Nor are we saying they cannot be used as a decision criterion in certain specialized applications (such as determining whether a manufacturing process meets some quality-control standard). And we are also not advocating for an anything-goes situation, in which weak evidence suddenly becomes credible. Rather, and in line with many others over the decades, we are calling for a stop to the use of P values in the conventional, dichotomous way – to decide whether a result refutes or supports a scientific hypothesis.
What does this mean for knowledge management?
The use of research evidence is a critical aspect of evidence-based knowledge management (KM). For effective evidence-based practice, knowledge managers need to be able to draw on research that isn’t hyped and that has thoroughly considered all potentially crucial effects. This means that calls for statistical significance to be retired are in the interests of better evidence-based KM.
Header image: Surveys of hundreds of articles have found that statistically non-significant results are wrongly interpreted as indicating ‘no difference’ or ‘no effect’ in around half. Source: V. Amrhein et al., © Nature.
References:
Also published on Medium.