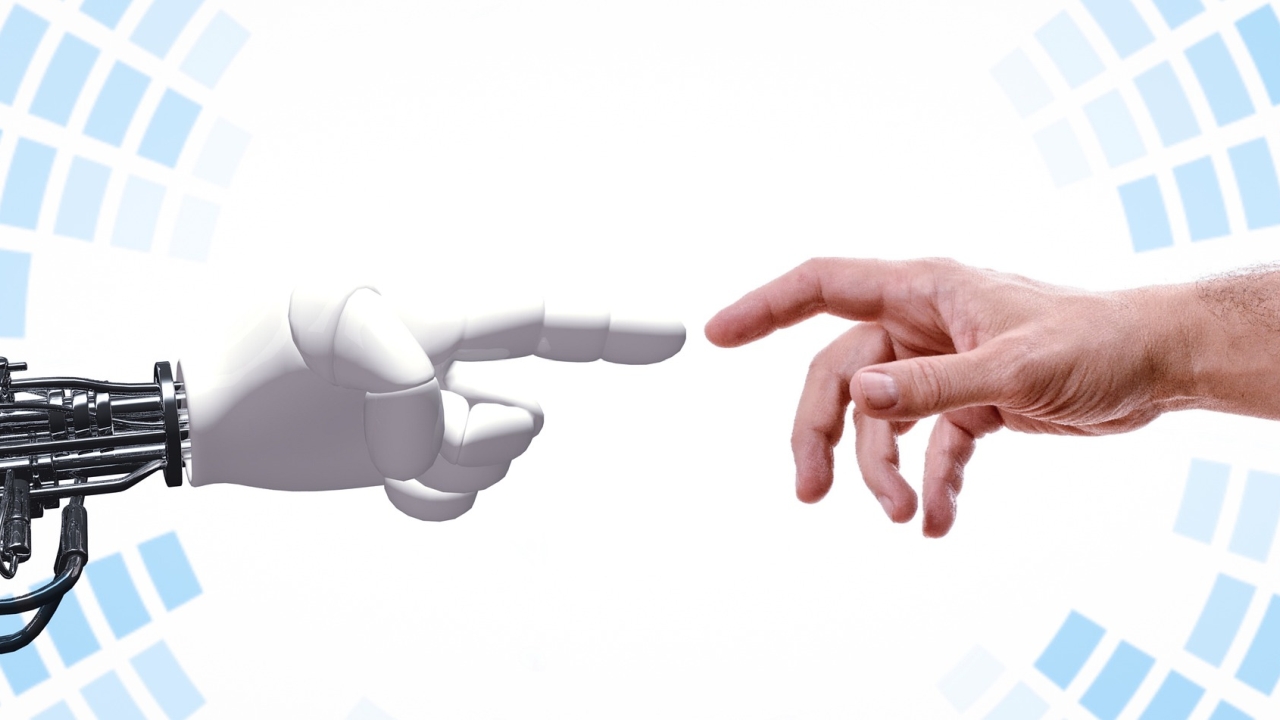
Combining human and artificial intelligence for organizational decision-making under uncertainty
Strategic organizational decision-making in today’s complex world is a dynamic and challenging knowledge management (KM) process characterized by uncertainty. Diverse groups of employees need to deal with a large amount and wide variety of internal and external information and knowledge, and this must be acquired and interpreted appropriately to determine adequate alternatives.
Artificial intelligence (AI) is expected to offer support to strategic organizational decision-making under uncertainty. Using a systematic literature review and content analysis, a recent study1 carries out what its authors consider is the first assessment of the current status of research in this regard.
The systematic literature review involved a keyword search of academic databases followed by evaluation against inclusion and exclusion criteria. This resulted in the identification of 55 articles published in 42 different academic journals. The content analysis was then carried out, which included assigning articles to six different identified categories.
The six categories, with a brief summary of the findings of the content analysis for each, are:
- KM with the help of AI – Researchers agree that AI can be used for the collection, interpretation, evaluation, and sharing of explicit information, thereby providing support in regard to speed, amount, diversity, and availability. Some researchers also offer potential tools to facilitate the availability of implicit information (which the KM community refers to as tacit knowledge).
- Categorization of AI applications – The choice of application is influenced by the various dimensions of data and the basic reason for which the technology is intended to be used. Most applications referred to in the reviewed research can be clustered as top–down, as they are not able to act self-consciously. However, with increasing research and development the capabilities of AI applications are expected to increase.
- Impact of AI on organizational structures – Organizational structures are the foundation for successful AI integration, and conversely, the use of AI in decision-making also influences those structures. The strategic reasons for implementing AI inform the type and location of AI used. However, the available applications are also expected to influence existing decision-making processes that are to be adapted to make usage possible.
- Challenges of using AI in strategic organizational decision-making – To determine whether, how, and why to integrate AI into existing business processes, AI literacy has been found to be crucial. Therefore, according to the reviewed research, education and training constitute a highly important task. The involvement of all employees who will be affected by AI integration, rather than just top management, is also considered crucial, as are transparency and the step-by-step introduction of AI applications. Additionally, issues related to data security, privacy, and manipulation must be addressed. Data manipulation includes the manifestation of biases.
- Ethical perspectives on using AI in strategic organizational decision-making – Although all researchers in this category state that an ethical framework is needed to use AI in organizational decision-making, there is no agreement on the design. As no clear recommendation can be derived on how to solve this challenge, it is suggested that managers actively engage employees and stakeholders is a process to agree on ethical guidelines.
- Impact of AI usage in strategic organizational decision-making on the division of tasks between humans and machines – The reviewed research claims that AI offers the potential for machines to augment human capabilities. At the same time, it also changes the human role to become more of a supervisor. Researchers expect that the potential for AI to be integrated into strategic organizational decision-making will be rather limited because capabilities are needed that only humans are argued to possess.
Conceptual model
From the content analysis, the study authors have developed the conceptual model in Figure 1. As shown in the model. the study authors have used the content analysis findings to identify a division of tasks between humans and AI for each step in the strategic organizational decision-making process.

The strategic organizational decision-making process proposed in the model is based on decision theory and several studies on decision-making under uncertainty. The process begins with the definition of the decision goal as the guideline for all subsequent steps. The information that must be collected in step two can be categorized as either external or internal, and either explicit (for example, facts and figures on the organization) or implicit (for example, employee experience).
Since decision-makers can only interpret information that is available, the quality and completeness resulting from step two influences the rest of the process. The amount of information also has an impact on the process, with organizations typically collecting large amounts of information that isn’t needed, but having limited capacity to process that information.
Knowledge flow from steps two and three continuously influences all further steps. Alternatives are determined in step four, for which probability and utility values are then assigned in step five. Finally, in step six, the group weighs the alternatives and makes the decision. In an ideal world, the resulting outcome matches the desired goal.
A concern
The conceptual model in Figure 1 is a very valuable first effort in identifying the division of tasks between humans and AI in the strategic organizational decision-making process. However, a concern that I have in regard to the conceptual model is that it represents the decision-making process as being linear, despite the authors having identified that the decision-making takes place in complex contexts.
As Ramalingam and colleagues discuss in the RealKM Magazine Exploring the science of complexity series2, one of the key concepts of complexity science is nonlinearity. In part 13 of the series, they state that:
complexity science suggests that human systems do not work in a simple linear fashion. Feedback processes between interconnected elements and dimensions lead to relationships that see change that is dynamic, nonlinear and unpredictable. Nonlinearity is a direct result of the mutual interdependence between dimensions found in complex systems. In such systems, clear causal relations cannot be traced because of multiple influences.
In response, I recommend that further research is carried out to address nonlinearity in the conceptual model and identify the implications of this for the division of tasks between humans and AI.
Article source: Trunk et al. 2020, CC BY 4.0.
Header image source: Mohamed Hassan on Pixabay, Public Domain.
References:
- Trunk, A., Birkel, H., & Hartmann, E. (2020). On the current state of combining human and artificial intelligence for strategic organizational decision making. Business Research, 13(3), 875-919. ↩
- Ramalingam, B., Jones, H., Reba, T., & Young, J. (2008). Exploring the science of complexity: Ideas and implications for development and humanitarian efforts (Vol. 285). London: ODI. ↩
Also published on Medium.