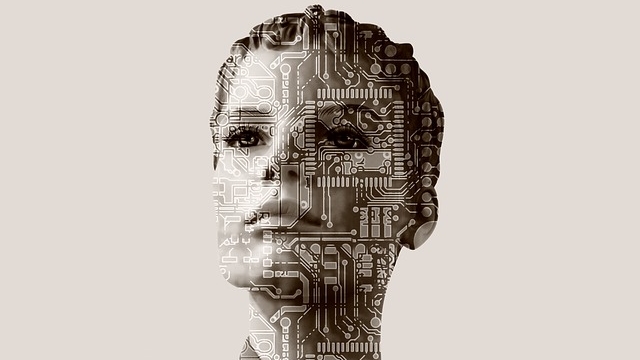
The questions that reveal how machines think
Originally posted on The Horizons Tracker.
The Turing Test famously aims to test the abilities of artificial intelligence by tasking humans with uncovering when they’re talking to a person and when they’re talking to a machine. It tests the ability of AI to understand human language sufficiently to be able to hold natural seeming conversations.
As anyone who has tried to have a conversation with a AI-powered chatbot or virtual assistant can attest, there remains some way to go before technology can master this most human of abilities. New research1 from the University of Maryland aims to help AI progress by identifying some 1,200 questions that while pretty easy for humans to answer, have traditionally stumped the best technology available today.
“Most question-answering computer systems don’t explain why they answer the way they do, but our work helps us see what computers actually understand,” the researchers explain. “In addition, we have produced a dataset to test on computers that will reveal if a computer language system is actually reading and doing the same sorts of processing that humans are able to do.”
Smarter machines
The researchers explain that many of the Q&A systems in operation today rely on either humans or computers to generate the questions that are designed to train the systems. The problem with this approach is that it’s not easy to understand why the computers are struggling to answer the questions correctly. The researchers believe that by better understanding what stumps the machines, we can better design datasets to train them.
The team developed a system that was capable of showing its thought processes as it attempted to answer each question, which they believe would not only give insight into the processes the computer was going through, but if deployed in a live environment, allow the human questioner to modify their line of enquiry.
The partnership between man and machine enabled 1,213 questions that had defeated the computer on its own to be successfully answered.
“For three or four years, people have been aware that computer question-answering systems are very brittle and can be fooled very easily,” the authors explain. “But this is the first paper we are aware of that actually uses a machine to help humans break the model itself.”
The team believe that the questions will serve as a valuable dataset to better inform work in natural language processing, while also acting as a training dataset, especially as the questions uncovered six distinct phenomena that baffled AI-based systems.
These failures emerged in either linguistic areas such as paraphrasing or unexpected context, or a failure in reasoning skills, such as the triangulation of the various elements in a question or the requirement to use multiple steps when forging a conclusion.
“Humans are able to generalize more and to see deeper connections,” Boyd-the researchers explain. “They don’t have the limitless memory of computers, but they still have an advantage in being able to see the forest for the trees. Cataloguing the problems computers have helps us understand the issues we need to address, so that we can actually get computers to begin to see the forest through the trees and answer questions in the way humans do.”
Suffice to say, there is a little way to go before this kind of scenario emerges, but the research is an interesting indication of the progress being made in enabling machines to better navigate the nuances of human language.
Article source: The Questions That Reveal How Machines Think.
Header image source: Gerd Altmann on Pixabay, Public Domain.
Reference:
- Wallace, E., Rodriguez, P., Feng, S., Yamada, I., & Boyd-Graber, J. (2018). Trick Me If You Can: Human-in-the-loop Generation of Adversarial Examples for Question Answering. arXiv preprint arXiv:1809.02701. ↩