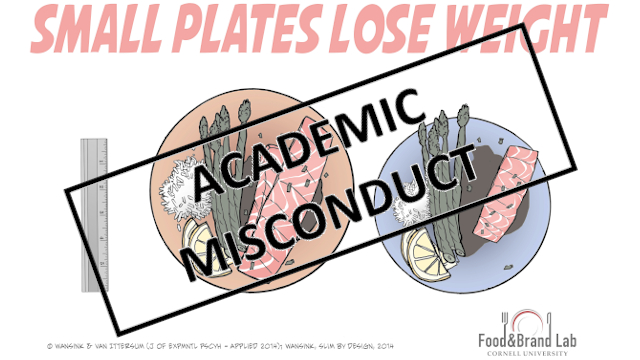
Simplistic solutions to complex problems turns behavioural science into a dangerous pseudoscience (part 2): Why it’s pseudoscience
This three-part series critiques the research of discredited high-profile Cornell University food researcher Brian Wansink from a complexity point of view.
In the first part of this series, I look at the deeper issue underlying the recent academic misconduct finding against high-profile Cornell University food researcher Brian Wansink.
While Wansink had misreported research data and employed problematic statistical techniques, including engaging in p-hacking, I argue that his use of these practices is actually a symptom of the true underlying problem, rather than the problem in itself. The real problem is that Wansink put forward simplistic solutions that ignored the complex reality of the health issues he was seeking to address, and the only way he could get these solutions to appear to work was through engaging in inappropriate practices. However, I don’t think that Wansink set out to intentionally do the wrong thing, but rather that continually reinforced confirmation bias blinded him to the complexity.
In this second part of the series, I look at how advancing simplistic solutions in ignorance of complexity and compounding this ignorance through confirmation bias turns behavioural science into a pseudoscience.
Why it’s pseudoscience
An examination of how advancing simplistic solutions in ignorance of complexity and compounding this ignorance through confirmation bias turns behavioural science into a pseudoscience needs to begin with defining pseudoscience.
While there’s philosophical debate1 in regard to the demarcation between science and pseudoscience, pseudoscience is seen as being incompatible with the scientific method and having a number of key characteristics. The Wikipedia entry on pseudoscience provides the following definition and list of characteristics:
Pseudoscience consists of statements, beliefs, or practices that are claimed to be both scientific and factual, but are incompatible with the scientific method. Pseudoscience is often characterized by contradictory, exaggerated or unfalsifiable claims; reliance on confirmation bias rather than rigorous attempts at refutation; lack of openness to evaluation by other experts; and absence of systematic practices when developing theories, and continued adherence long after they have been experimentally discredited.
This definition has found support in the academic literature. For example, in the recent editorial “Science, pseudoscience, evidence-based practice and post truth” 2, Associate Professor José M. González-Méijome states that:
we should reintroduce the definition of pseudoscience according to the Oxford Dictionary as “a collection of beliefs or practices mistakenly regarded as being based on scientific method”. Therefore, pseudoscience arises when those sources of knowledge assume the role of science itself. The reader might find it helpful to recognise pseudoscience by some of its characteristics according to some authors: used to be contradictory, makes exaggerated or unprovable claims, relies on confirmation bias rather than rigorous attempts at refutation, lack of openness to evaluation by other experts, and absence of systematic practices when developing theories.
So while mention of the word pseudoscience may immediately bring to mind “out there” topics such as the paranormal and parapsychology, the definition also encompasses things that can be inappropriate practices in mainstream science: a reliance on confirmation bias rather than rigorous attempts at refutation and an absence of systematic practices when developing theories.
In part 1 of this series, I highlighted the significant complexity of the health issues such as obesity that Wansink’s research sought to address. In the article “Learning from Evidence in a Complex World” 3 in the American Journal of Public Health, Professor John D. Sterman alerts that:
Generating reliable evidence through scientific method requires the ability to conduct controlled experiments, discriminate among rival hypotheses, and replicate results. But the more complex the phenomenon, the more difficult are these tasks. Medical interventions and health policies are embedded in intricate networks of physical, biological, ecological, technical, economic, social, political, and other relationships. Experiments in complex human systems are often unethical or simply infeasible … Replication is difficult or impossible … Decisions taken in one part of the system ripple out across geographic and disciplinary boundaries. Long time delays mean we never experience the full consequences of our actions. Follow-up studies must be carried out over decades or lifetimes, while at the same time changing conditions may render the results irrelevant. Complexity hinders the generation of evidence.
In the comprehensive 2013 review “Scientific Decision Making, Policy Decisions, and the Obesity Pandemic” 4 in Mayo Clinic Proceedings, a group of researchers from the University of South Carolina, University of Alabama at Birmingham, and University of Queensland provide what amounts to a damning assessment of the simplistic approach that Wansink has taken to this complexity.
As I discussed in part 1 of this series, a key solution to chronic health issues including obesity that was advanced by Wansink was the food environment change of reducing portions size, as shown in the Food & Brand Lab image above.
The authors of “Scientific Decision Making, Policy Decisions, and the Obesity Pandemic” state that:
- “intersecting shortcomings [in addressing obesity include] … (2) a range of methodologic issues that encourage generating data that perpetuate misconceptions about obesity’s causes, and (3) scholarly dialogues, including peer review processes, that uncritically accept a priori assumptions about cause derived from inaccurate models of obesity and inadequate evidential foundations”
- “Simplistic notions derived from the [first law of thermodynamics] have led to numerous naive speculations regarding the obesity pandemic and interventions that ignore physiologic and behavioral compensation”
- “Recently, several articles have pointed out that there are many unproven beliefs (presumptions) and disproven beliefs (myths) about obesity erroneously circulating as facts not only in the mass media and the general public but also in the scientific community”
- “Example[s] of presumptions erroneously accepted as fact [include that] Reducing portion size in some sources of food will reduce body weight or lead to less weight gain in the long-term.”
They go on to highlight how these simplistic presumptions erroneously accepted as fact are reinforced by crude models, confirmation bias, and ignorance of complexity:
Crude models of obesity have constrained research for decades, from study design choices, selection and measurement of variables, operational definitions, development and use of measurement tools, and decisions as to how, when, and where to intervene. The flawed logic, vague concepts, inaccurate and imprecise measurements, and unsubstantiated a priori assumptions regarding causal relationships that have followed from vague and poorly specified models during the past century have engendered policy and funding decisions that have incentivized research that perpetuates and compounds these errors via confirmation bias while constraining research paths incongruent with prevailing assumptions.
Conjectures emanating from studies based on the current models of obesity coupled with policy and funding decisions that narrowly constrain future inquiry have contributed to a failure of the peer review process to deepen understanding of obesity at all levels of inquiry. To understand the scope of the problem, we need to remain aware of the fact that obesity arises from the dynamic interplay of the external environment with behavioral and developmental processes and genetic and epigenetic factors. When conceptualized in this manner, it becomes evident that simple, deterministic statements about the etiology of obesity and narrow interpretation about the scope of fundable research are naive and inherently unscientific.
However, this isn’t just “inherently unscientific” as the authors conclude. The conduct of “incentivized research that perpetuates and compounds these errors via confirmation bias while constraining research paths incongruent with prevailing assumptions” matches the “reliance on confirmation bias rather than rigorous attempts at refutation” aspect of the earlier definitions of pseudoscience. Similarly, the authors’ statements in regard to how “Crude models of obesity have constrained research for decades …” match the “absence of systematic practices when developing theories” aspect of the earlier definitions of pseudoscience.
So, on the basis of the evidence, advancing simplistic solutions in ignorance of complexity and compounding this ignorance through confirmation bias turns behavioural science into a pseudoscience.
In the forthcoming third part of the series, I’ll show that this is dangerous because of the negative consequences that result from simplistic solutions to the complex chronic health issue of obesity.
Next and final part (part 3): Why it’s dangerous.
Header image source: Small Plates Lose Weight is licensed under CC BY-NC-ND 4.0.
References:
- Hansson, S.O. (2017). “Science and Pseudo-Science”, The Stanford Encyclopedia of Philosophy (Summer 2017 Edition), Edward N. Zalta (ed.). ↩
- González-Méijome, J. M. (2017). Science, pseudoscience, evidence-based practice and post truth. Journal of Optometry, 10(4), 203. ↩
- Sterman, J. D. (2006). Learning from evidence in a complex world. American Journal of Public Health, 96(3), 505-514. ↩
- Hebert, J. R., Allison, D. B., Archer, E., Lavie, C. J., & Blair, S. N. (2013, June). Scientific decision making, policy decisions, and the obesity pandemic. In Mayo Clinic Proceedings (Vol. 88, No. 6, pp. 593-604). Elsevier. ↩
Also published on Medium.