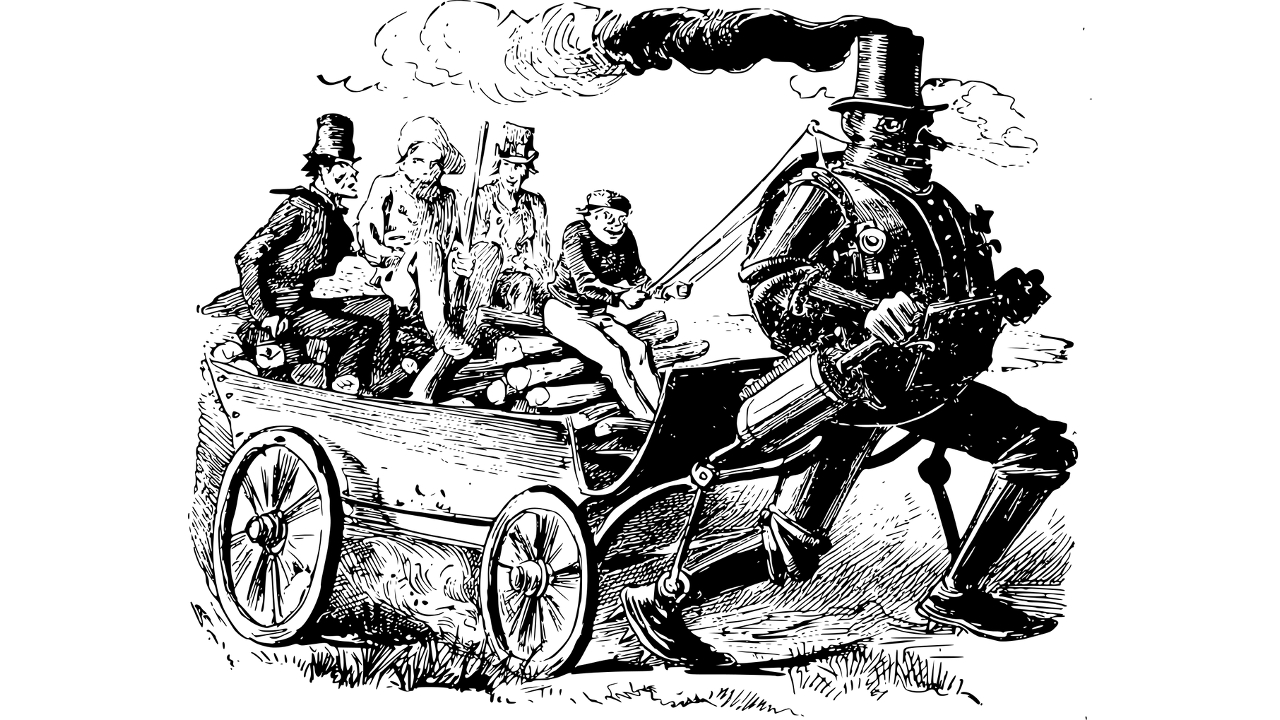
Decolonial AI: Decolonial Theory as Sociotechnical Foresight in Artificial Intelligence [Top 100 research & commentary of 2020]
This article is part 11 (and the final part) of a series reviewing selected papers and associated commentary from Altmetric’s list of the top 100 most discussed and shared research and commentary of 2020.
In the #87 article1 in Altmetric’s top 100 list for 2020, Shakir Mohamed, Marie-Therese Png, and William Isaac aim to provide a perspective on the importance of a critical science approach, and in particular of decolonial thinking, in understanding and shaping ongoing advances in artificial intelligence (AI).
Algorithmic coloniality
Mohamed, Png, and Isaac advise that, like physical spaces, digital structures can become sites of extraction and exploitation, and thus the sites of coloniality. The coloniality of power can be observed in digital structures in the form of socio-cultural imaginations, knowledge systems, and ways of developing and using technology which are based on systems, institutions, and values which persist from the past and remain unquestioned in the present. As such, emerging technologies like AI are directly subject to coloniality.
To describe this “algorithmic coloniality,” Mohamed, Png, and Isaac introduce a taxonomy of decolonial foresight: algorithmic oppression, algorithmic exploitation, and algorithmic dispossession. Within these forms of decolonial foresight, they present a range of use cases that they identify as sites of coloniality: algorithmic decision systems, ghost work, beta-testing, national policies, and international social development.
Algorithmic oppression
Algorithmic oppression extends the unjust subordination of one social group and the privileging of another – maintained by a “complex network of social restrictions” ranging from social norms, laws, institutional rules, implicit biases, and stereotypes – through automated, data-driven, and predictive systems.
Algorithmic exploitation
Algorithmic exploitation considers the ways in which institutional actors and industries that surround algorithmic tools take advantage of (often already marginalised) people by unfair or unethical means, for the asymmetrical benefit of these industries. The following examples examine colonial continuities in labour practices and scientific experimentation in the context of algorithmic industries.
Algorithmic dispossession
Algorithmic dispossession describes how, in the growing digital economy, certain regulatory policies result in a centralisation of power, assets, or rights in the hands of a minority and the deprivation of power, assets, or rights from a disempowered majority. The following examples examine this process in the context of international AI governance (policy and ethics) standards, and AI for international social development.
Tactics for a decolonial AI
In consideration of the five sites of coloniality above, Mohamed, Png, and Isaac propose sets of tactics for the future development of AI, which they believe open many areas for further research and action. They advise that tactics do not lead to a conclusive solution or method, but instead to the contingent and collaborative construction of other narratives.
Mohamed, Png, and Isaac submit three sets of tactics: supporting a critical technical practice of AI, establishing reciprocal engagements and reverse learning, and the renewal of affective and political community.
Towards a critical technical practice of AI
Critical technical practices (CTPs) take a middle ground between the technical work of developing new AI algorithms and the reflexive work of criticism that uncovers hidden assumptions and alternative ways of working. CTP has been widely influential, having found an important place in human-computer interactions (HCI) and design. By infusing CTP with decoloniality, a productive pressure can be applied to technical work, moving beyond good-conscience design and impact assessments that are undertaken as secondary tasks, to a way of working that continuously generates provocative questions and assessments of the politically situated nature of AI.
Mohamed, Png, and Isaac explore five topics constituting such a practice:
- Fairness. Efforts at fairness can still lead to discriminatory or unethical outcomes for marginalised groups, depending on the underlying dynamics of power; because definitions of fairness are often a function of political and social factors. There is a need to question who is protected by mainstream notions of fairness, and to understand the exclusion of certain groups as continuities and legacies of colonialism embedded in modern structures of power, control, and hegemony. This speaks to a critical practice whose recent efforts, in response, have proposed fairness metrics that attempt to use causality or interactivity to integrate more contextual awareness of human conceptions of fairness.
- Safety. The area of technical AI safety is concerned with the design of AI systems that are safe and appropriately align with human values. The philosophical question of value alignment arises, identifying the ways in which the implicit values learnt by AI systems can instead be aligned with those of their human users. There is a need to question whose values and goals are represented, and who is empowered to articulate and embed these values. Of importance here is the need to integrate discussions of social safety alongside questions of technical safety.
- Diversity. With a critical lens, efforts towards greater equity, diversity, and inclusion (EDI) in the fields of science and technology are transformed from the prevailing discourse that focuses on the business case of building more effective teams or as being a moral imperative, into diversity as a critical practice through which issues of homogenisation, power, values, and cultural colonialism are directly confronted. Such diversity changes the way teams and organisations think at a fundamental level, allowing for more intersectional approaches to problem-solving to be taken.
- Policy. There is growing traction in AI governance in developing countries to encourage localised AI development, or in structuring protective mechanisms against exploitative or extractive data practices. Although there are clear benefits to such initiatives, international organisations supporting these efforts are still positioned within the old colonial powers, maintaining the need for self-reflexive practices and considerations of wider political economy.
- Resistance. The technologies of resistance have often emerged as a consequence of opposition to coloniality. A renewed critical practice can also ask the question of whether AI can itself be used as a decolonising tool, e.g. by exposing systematic biases and sites of redress. Furthermore, although AI systems are confined to a specific sociotechnical framing, Mohamed, Png, and Isaac believe that they can be used as a decolonising tool while avoiding a techno-solutionism trap.
Reciprocal engagements and reverse learning
Despite colonial power, the historical record shows that colonialism was never only an act of imposition. In a reversal of roles, the colonists often took lessons from the colonised, establishing a reverse learning. Reverse learning directly speaks to the philosophical questions of what constitutes knowledge. Deciding what counts as valid knowledge, what is included within a dataset and what is ignored and unquestioned is a form of power held by AI researchers that cannot be left unacknowledged. It is in confronting this condition that decolonial science, and particularly the tactic of reverse learning, makes its mark.
Mohamed, Png, and Isaac put forward three modes through which reciprocal learning can be enacted:
- Dialogue. A decolonial shift that can be achieved by systems of meaningful intercultural dialogue. Such dialogue is core to the field of intercultural digital ethics, which asks questions of how technology can support society and culture, rather than becoming an instrument of cultural oppression and colonialism.
- Documentation. New frameworks have been developed that make explicit the representations of knowledge assumed within a dataset and within deployed AI systems.
- Design. There is now also a growing understanding of approaches for meaningful community-engaged research, using frameworks like the IEEE Ethically Aligned Design, technology policy design frameworks like Diverse Voices, and mechanisms for the co-development of algorithmic accountability through participatory action research. The framework of citizens’ juries have also been used to gain insight into the general public’s understanding of the role and impact of AI.
A critical viewpoint may not have been the driver of these solutions, and these proposals are themselves subject to limitations and critique, but through an ongoing process of criticism and research, they can lead to powerful mechanisms for reverse learning in AI design and deployment.
Renewed affective and political communities
How we build a critical practice of AI depends on the strength of political communities to shape the ways they will use AI, their inclusion and ownership of advanced technologies, and the mechanisms in place to contest, redress, and reverse technological interventions. The decolonial imperative asks for a move away from attitudes of technological benevolence and paternalism. The challenge lies in how new types of political community can be created that are able to reform systems of hierarchy, knowledge, technology, and culture at play in modern life.
One tactic lies in embedding the tools of decolonial thought within AI design and research. Contrapuntal analysis is one important critical tool that actively leads to exposing the habits and codifications that embed questionable binarisms in research and products. Another tactic lies in support of grassroots organisations and in their ability to create new forms of affective community, elevate intercultural dialogue, and demonstrate the forms of solidarity and alternative community that are already possible. Many such groups already exist, particularly in the field of AI, such as Data for Black Lives, the Deep Learning Indaba, Black in AI, and Queer in AI, and are active across the world.
The advantage of historical hindsight means that the principles of living that were previously made incompatible with life by colonial binaries can now be recovered. Friendship quickly emerges as a lost trope of anticolonial thought. This is a political friendship that has been expanded in many forms: in the politics of friendship and as affective communities in which developers and users seek alliances and connection outside possessive forms of belonging.
Finally, these views of AI taken together lead quickly towards fundamental philosophical questions of what it is to be human – how we relate and live with each other in spaces that are both physical and digital, how we navigate difference and transcultural ethics, how we reposition the roles of culture and power at work in daily life – and how the answers to these questions are reflected in the AI systems we build.
What does this mean for knowledge management?
Decolonial AI needs to be considered as an important aspect of the decolonisation of knowledge and knowledge management (KM). Further, some of the tactics for a decolonial AI put forward above have the potential to be applied not just to AI, but in the broader decolonisation of knowledge context, and this should also be considered,
Article source: Decolonial AI: Decolonial Theory as Sociotechnical Foresight in Artificial Intelligence, CC BY 4.0.
Header image source: OpenClipart-Vectors on Pixabay, Public Domain.
References:
- Mohamed, S., Png, M. T., & Isaac, W. (2020). Decolonial AI: Decolonial theory as sociotechnical foresight in artificial intelligence. Philosophy & Technology, 33(4), 659-684. ↩
Also published on Medium.