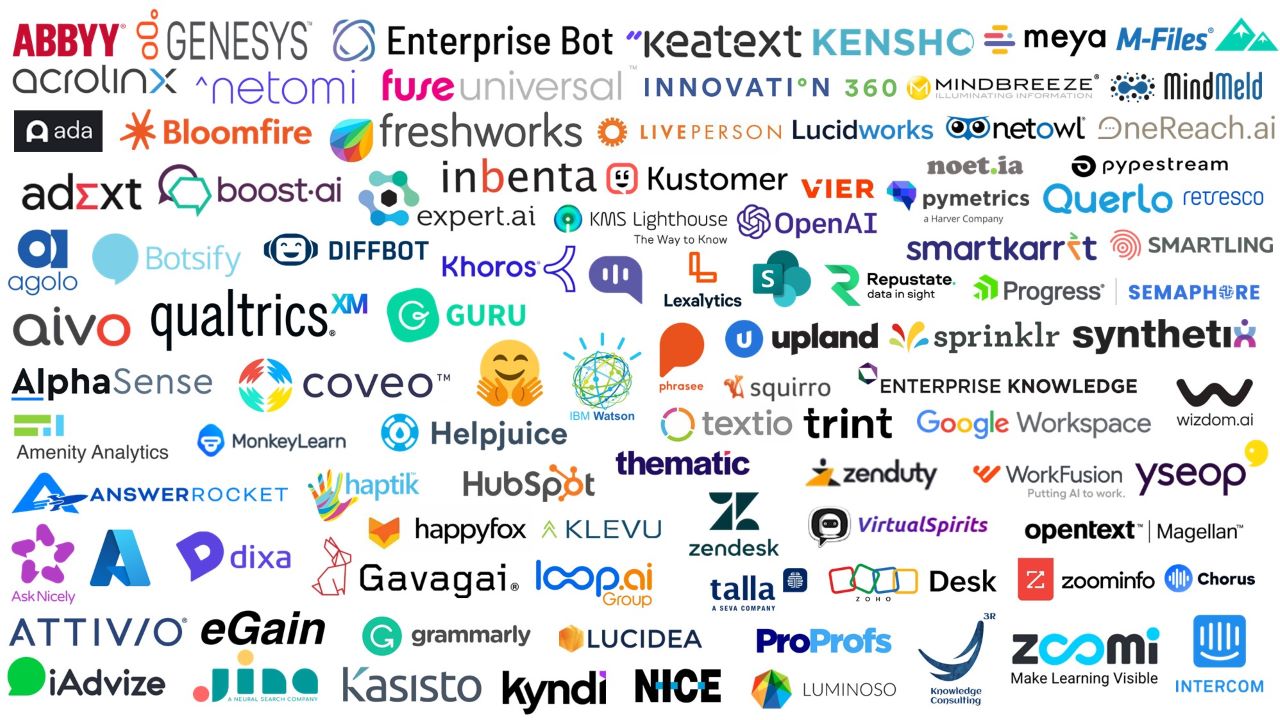
Summary and conclusion [Generative AI & KM series part 9]
This article is part 9 (and the final part) of the series AI integration strategy for learning and knowledge management solutions.
A comparative study of 100 generative AI tools in the context of learning and knowledge management (KM) was conducted and has resulted in a set of 35 KM processes where generative AI1 has augmented their experience, implementation, and execution. This article (part 9 and the final part in the series) provides the summary and conclusion of the study.
The upside
Generative AI can help in:
- personalizing the learning experience, providing assisted coaching, and suggesting skills for individual career growth
- curating and suggesting relevant learning materials in multiple languages, in addition to transcribing and translating audio & video content for almost instant access for the global audience
- drafting missing articles, reports, and creating knowledge portals
- augmenting the community experience by suggesting topical communities, matching mentor-mentee pairs, enriching the sharing behaviors with sister communities, and generating knowledge narratives and stories
- accelerating the ideation and creativity processes by mapping and connecting ideas and idea authors to innovation campaign objectives
- creating rich content either by drafting missing knowledge base articles or by auto-completing ideas with arguments and examples
- searching and extracting answers from specific document sections after analyzing the context, the sentiment, and the intent of the user query
- improving human-in-the-loop collaboration by suggesting experts based on their activities, involvement, and preferences
- inferring expertise and micro-skills by analyzing content authors and contributors’ behaviors and patterns of engagement, and as consequence, assist in augmenting peoples’ profiles with micro-skills and topics of interests
- automating, extracting, and regenerating knowledge into new formats or schemas
- extracting competitor’s websites and all the spider’s links into a structured data list with their properties and meta-data
- enriching content with meta-data and semantic relationships and generating a text into a knowledge graph heavily relying on natural language processing (NLP)
- impacting the customer’s experience by offering self-service portals, FAQs or recommended relevant content making the customer experience more conversational and interactive
- integrating with multi-modal systems to scale the data infrastructure for a more comprehensive and integrated database by linking it to different sources of information
- automating the data migration process by removing duplicate entries or by combining similar data.
AI has introduced the concept of digital worker who’s a human like clone for specific roles, responsibilities, and tasks. Digital workers are characterized by being curious, collaborative, and capable. Digital workers can be pre-loaded with smart skills which are signature patterns and workflows replicating industry-specific practices.
The downside
AI algorithms require guidance and supervision to define which information and data are most important to the users to lay down the foundation for meaningful insights and best/ direct answers.
It’s important to note that while large language models (LLMs) like GPT-3 are powerful in generating text, they are not inherently intelligent or conscious. They don’t possess an original understanding or awareness of the content they generate and may sometimes produce outputs that are nonsensical or inappropriate. Therefore, they require human supervision – known as reinforced learning and careful application to ensure their outputs meet the desired outcome.
The cost of implementing an internal infrastructure for generative AI / LLM technology deployment can rise significantly based on factors such as the scale of deployment, the complexity of the technology, the size of the organization, and the specific requirements of the project. Additionally, if an organization decides to use cloud based LLM cognitive services, concerns around governance, security and privacy will be a legitimate subject to carefully consider.
Further reading: This article is the final part in the series AI integration strategy for learning and knowledge management solutions. For further reading related to this topic, please see the ongoing artificial intelligence series.
Header image source: Author provided.
Reference:
- Najjar, R. (2023, July 13). Preliminary Understanding of Generative AI: What & How? Medium. ↩
Can I receive your newsletter please ? Thanks
Thank you for your interest in the RealKM Weekly Wrap e-newsletter. To subscribe, please visit https://realkm.com/subscribe-to-e-news/